Citation
De Martino, M., Morvillo, A. and Giordano, G. (2024), "Social network analysis in hospitality and tourism: Guest editorial", International Journal of Contemporary Hospitality Management, Vol. 36 No. 2, pp. 349-357. https://doi.org/10.1108/IJCHM-02-2024-161
Publisher
:Emerald Publishing Limited
Copyright © 2023, Emerald Publishing Limited
Introduction
Social network analysis (SNA) has rapidly grown in the hospitality and tourism field in the past two decades. Although this approach remains widely applied in bibliometric studies, shedding light on the intellectual structure, progress of scientific collaboration and research themes, other areas of increasing interest concern the study of collaboration and stakeholders’ interactions as vehicles of innovation, sustainable development and value co-creation processes. The special issue aims to provide theoretical, methodological and empirically relevant contributions to the study of hospitality and tourism through the lenses of the SNA. It includes 13 studies that address a variety of topics and perspectives, embracing quantitative, qualitative and mixed approaches of analysis.
To present an overview of the SI’s contents, we exploit textual definitions given in the 13 abstracts as reported by authors and carry out a text mining approach. The abstracts have been considered along four dimensions: purpose, methodology, findings and keywords. For each dimension, the corpus has been analyzed by the “tm” package in R (Feinerer and Hornik, 2022), and texts have been lemmatized to produce four lexical matrices (terms per papers). In the context of SNA, these four matrices can be read as affiliation matrices defining bipartite networks. In a bipartite network, there exist two sets of nodes, in our case, the set of lemmas and the set of papers. Edges are defined only among the nodes belonging to different sets. The resulting graphs give evidence of connections among each paper and the most representative lemmas as defined in the lexical matrices.
On each bipartite network, the Louvain (Blondel et al., 2008) community detection method has been produced. The community detection algorithm allows determining those sub-graphs where there are more dense relationships among nodes inside a community, while the different communities show less dense ties. Computation has been carried out by the “igraph” package in R (Csardi and Nepusz, 2006).
In the next section, we present a summary of the papers included in the special issue, regrouped according to the main areas of contribution: business networks, innovation and sustainability; social media branding strategies; network proprieties and structural characteristics; social capital and risks; and research trends and scientific collaboration. Additionally, to offer alternative keys to readers, the community detection method on abstract description has been carried out. Conclusions are provided in the last section.
Business networks, innovation and sustainability
McLeod et al. (2022) examined the information flows and knowledge sharing between managers and owners in a tourism destination in South-West England and their contribution to innovation. Results indicate that social network characteristics impact differently on business practices innovation.
Blasi et al. untangled the role of cognitive and geographical proximity in the formation of sustainable tourism business networks in northeastern Italy. Tourism operators in peripherical areas are often more committed to sustainability and collaborate with other destinations to create a sustainable ecosystem. Wang et al. (2022) explored social networks and network interactions of bed-and-breakfast entrepreneurs in rural China. Three typologies of business network – business operation, business development and business citizenship – can determine entrepreneurs’ interactions with social actors and influence on business, community as well as rural resilience.
Social media branding strategies
Wang et al. (2023) explored brand formation and diffusion processes in World Heritage Sites’ in Beijing through a mixed method which connects text mining, keyword analysis and SNA. The study contributes to the understanding of social media branding mechanism which includes brand diffusion, user-generated content, opinion leaders and brand events’ impact.
Breznik et al. (2022) investigated the content of hotel brands’ mission statements and their relationship with hotel brands attributes. Results show differences between luxurious and midscale category hotel brands, in selecting keywords and attributes to communicate in marketing strategies.
Vila-Lopez and Kuster-Boluda (2022) investigated the evolution of the image of a religious tourism destination (Vatican City) before and since the pandemic crisis. The search of spiritual consolation in a global health crisis determines the repositioning brand strategy of the destination based on a mix of spiritual, cultural and relaxation experiences.
Network proprieties and structural characteristics
Ness et al. (2022) investigated how and to what extent structural network properties affect dyadic negotiation behavior in tourism destinations in Southeastern Norway. Dyad’s structural network embeddedness has implications for negotiation behavior and that triadic closure and relationship duration have positive effects on problem-solving.
Baggio et al. (2022) examined interlocking directorates among hotel corporations whose headquarters are in top Italian tourism destinations. The low number of interlocks between people (board members) and corporations’ (hotels) networks leads a fragmented and dispersed structure of the Italian hospitality industry.
Ivars-Baidal et al. (2023) examined interconnectedness between tourism governance and smart city development from a stakeholder perspective. The analysis reveals a disconnection between tourism and smart policies in most European cities under investigation, lacking collaborative structures and a broad social participation.
Chen (2022) aimed to model tourist activity according to the network approach, to explore its properties and to understand tourism consumption, supply and destination competitiveness. Using Hong Kong inbound tourist data, the study contributes from a macroscopic angle by examining network topology and from a microscopic angle by examining node centralities.
Social capital and risks
Jokar et al. (2022) investigated social risks in construction projects implemented in densely populated urban area hosting cultural heritage and historic tourism destination in Iran. The analysis shows that the most important social risks in the construction projects of the case study are “Psychological disorders”, “Environmental pollution” and “Cultural conflicts”.
Shrestha et al. (2023) explored how grassroots associations of indigenous women tourism entrepreneurs, from the rural Manasalu region of Nepal, can leverage their social network to co-create value. Social capital and support ensure sustenance and stability of grassroots associations, through collective and continuous learning.
Research trends and scientific collaboration
Yang et al. (2022) explored research interests and trends in co-authorship, co-citation and keyword co-occurrence in the fields of tourism and the pandemic. The study proposes a knowledge map of the leading articles and provides potential for future tourism research, especially in the global pandemic crises.7
The characterization of the thirteen papers by text mining and social network analysis
The studies in this special issue have different methodologies, findings, implications and topics as emerging from the keywords. We exploit these aspects held in each paper’s abstract to build a textual database. We present the results of a textual data analysis carried out on each of these dimensions. The 13 articles and the 4 features (methodologies, findings, implications and keywords) define a multidimensional corpus. Each dimension has been individually analyzed to give a different insight on the papers and to furnish different keys of understanding so helping the readers in finding an autonomous path across the Special Issue.
The text mining approach consists in building the document/term matrix: a quite large and sparse matrix holding all the terms (hundreds of columns) and documents (here we have 13 rows). In this view, the bag-of-words model allows to represent each document as a high-dimensional vector whose elements represent the importance of each term in each document. Usually, the importance is represented by the frequency of the term in the document; we normalize the frequencies applying the term frequency-reciprocal document frequency (TF-IDF), the term frequency–inverse document frequency (Salton and Buckley, 1988). TF-IDF is a weighting system that assigns a weight to each word in a document based on its TF and the IDF.
The words with higher scores of weight are deemed more significant. The resulting weighted document-term matrix has been used here to define a weighted bipartite network. In a bipartite network, there are two node sets (namely, terms and documents), and edges are allowed only between the two sets. In our analysis, an edge connects a term with a document, and it is weighted according to the importance of the term in the document. Considering separate analyses for each of the four abstract features, we have built four bipartite networks for the word-sets used to describe the methodology, the findings, the implications and the keywords.
In the following, we show the graph representation for each bipartite network with two different sets of symbols: circle for terms and square for documents (labeled with a number from 1 to 13) [1]. For each network, the Louvain community detection algorithm (Blondel et al., 2008) allows to detect non-overlapping subgraphs being densely connected internally and having sparser connections between them. Each community is represented by different colors in each graph representations, sharing a common subset of representative terms reported in the tables.
As it is evident from the graphs and tables, only a few papers show a tendency to appear in the same community along the different features such as (8–13) or (5–7). This indicates that descriptions provided by authors offer a heterogeneous picture but that somehow echo a common methodological root. In this regard, the bipartite textual network shows more densely internally connected communities in the methodology with respect to the other features. There are four communities based on common representative terms, one of which is composed of five papers (light blue) with a central position in the network (figure and table 1). In this community, the most frequently used words are “destinations and networks”, which clearly are related to the areas of investigation of the papers: “Network proprieties and structural characteristics”. Other communities show a common subset of representative terms, such as “text and mining” in papers 9–10 (green) and “social, actors, interviews” in papers 6–8–12 (yellow), although with sparser connections between them.
With reference to Findings, there are less densely internally connected communities but with some representative and common terms: papers 4–8–13 (light blue) investigate network structure, activities, and positive (effects); papers 3–10 (yellow) analyze the crisis’ (pandemic) impacts on city (Vatican) and paper (publication). Finally, the bipartite textual network Implications shows common subsets of representative terms such as managers and policy makers in the light blue community and destination in the orange; while, there are very few and sparse connections in the feature Keywords.
Concluding remarks
This special issue offers a comprehensive overview of the study of hospitality and tourism according to the SNA. Embracing a wide array of topics, this special issue sheds light on the main areas of contribution: business networks, innovation and sustainability; social media branding strategies; network proprieties and structural characteristics; social capital and risks; and research trends and scientific collaboration. Additionally, based on the community detection method, the 13 papers have been regrouped according to word sets used to describe the methodology, the findings, the implications and the keywords. Although heterogeneous, contributions echo a common methodological root. In this regard, we would offer to the readers further insights with respect to the nature of the approaches of analysis of the papers included in this Special Issue. Most of the papers adopt quantitative methods, including statistical analyses and observational data (2–3–4–5–9–10–11–13). Few papers (6–7–8–12) use qualitative approaches of analysis while only one (1) designed mixed approaches to examine social networks. In line with Mariani and Baggio (2020), we would encourage future research to embrace mixed methods of analysis, such as explanatory or exploratory sequential designs, to get a deeper understanding of networks in hospitality and tourism. In conclusion, this special issue collectively advances our knowledge of SNA in hospitality and tourism and provides valuable insights for both industry practitioners and researchers alike.
Figures
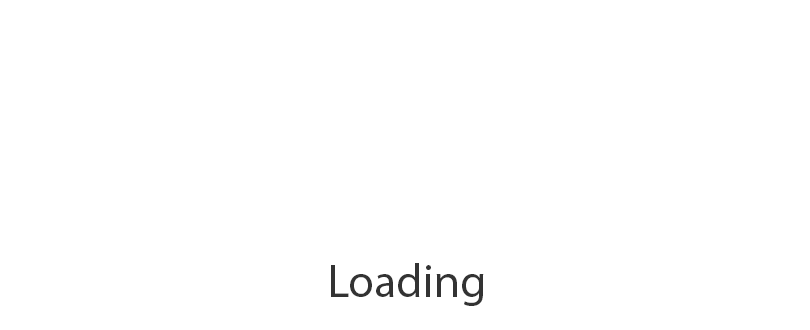
Figure 2.
The bipartite textual network and its communities – feature: Findings (see Table 2)
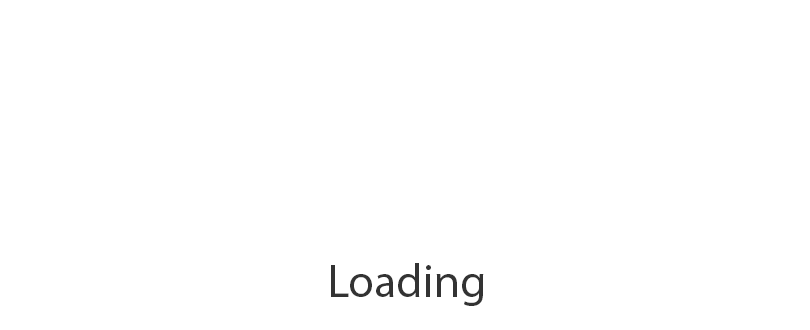
Figure 3.
The bipartite textual network and its communities – feature: Implications (see Table 3)
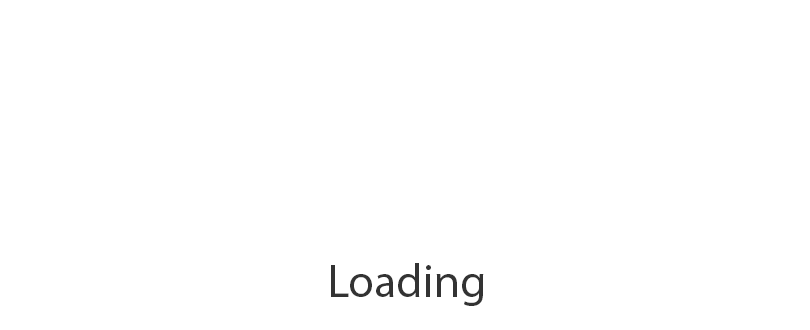
Figure 4.
The bipartite textual network and its communities – feature: Keywords (see Table 4)
The bipartite textual network and its communities – feature: methods
Community | Documents | Representative terms |
---|---|---|
1 – Yellow | 6–8–12 | Social; tourism; actors; interviews; owners;… |
2 – Light Blue | 1–4–5–7–13 | Destination/s; network/s; study; nodes; model; mechanism; … |
3 – Green | 9–10 | Text; mining; software; Web; … |
4 – Orange | 2–3–11 | Analysis; used; authors; hotel; stakeholders; … |
Source: Authors’ own creation
The bipartite textual network and its communities – feature: findings
Community | Documents | Representative terms |
---|---|---|
1 – Yellow | 3–7–10 | Tourism; crisis; governance; city; cultural; … |
2 – Light orange | 1–12 | Pandemic; interaction/s; influence; business; … |
3 – Light blue | 4–8–13 | Tourist; structure; activities; positive; networks; dissemination; information; |
4 – Orange | 9 | network; strong; sustainable; … |
5 – Blue | 2–6 | Social; support; … |
6 – Green | 5–11 | High; dimensions; degree; hospitality; … |
Source: Authors’ own creation
The bipartite textual network and its communities – feature: implications
Community | Documents | Representative terms |
---|---|---|
1 – Yellow | 7–11 | Understanding; urban; relationships; efficiency; … |
2 – Light Orange | 1–8–10–13 | Network; brand; different; destinations; tourism; … |
3 – Light Blue | 2–3–9 | Managers; policymakers; collaborations; … |
5 – Blue | 6 | Social; creation; knowledge; … |
6 – Green | 4–5–12 | Study; interaction; networks; business; … |
Source: Authors’ own creation
The bipartite textual network and its communities – feature: keywords
Community | Documents | Representative terms |
---|---|---|
1 – Yellow | 6–9–10 | Tourism; data; mining; city; … |
2 – Light orange | 1–2 | Urban; heritage; sites … |
3 – Light blue | 5–7–11 | Social; analysis; … |
5 – Blue | 3 | Knowledge; sustainable; … |
6 – Green | 4–8–12–13 | Network/s; structural; destinations; … |
Source: Authors’ own creation
Note
These are the numbers associated to the 13 papers: 1 – Wang et al. (2023); 2 – Jokar et al. (2022); 3 – Yang et al. (2022); 4 – Ness et al. (2022); 5 – Baggio et al. (2022); 6 – Shrestha et al. (2023); 7 – Ivars-Baidal et al. (2023); 8 – McLeod et al. (2022); 9- Blasi et al. (2022); 10 – Vila-Lopez and Kuster-Boluda (2022); 11 – Breznik et al. (2022); 12 – Wang et al. (2022); 13 – Chen (2022).
References
Baggio, R., Guizzardi, A. and Mariani, M. (2022), “A social network analysis of interlocking directorates in the accommodation sector”, International Journal of Contemporary Hospitality Management, doi: 10.1108/IJCHM-03-2022-0315.
Blasi, S., Fano, S., Sedita, S.R. and Toschi, G. (2022), “A network perspective of cognitive and geographical proximity of sustainable tourism organizations: evidence from Italy”, International Journal of Contemporary Hospitality Management, doi: 10.1108/IJCHM-03-2022-0366.
Blondel, V.D., Guillaume, J.-L., Lambiotte, R. and Lefebvre, E. (2008), “Fast unfolding of communities in large networks”, Journal of Statistical Mechanics: Theory and Experiment, Vol. 2008 No. 10, p. 10008.
Breznik, K., Zupan Korže, S., Ragozini, G. and Gorenak, M. (2022), “Revealing mission statements in a hotel brand sector using social network analytic approach”, International Journal of Contemporary Hospitality Management, doi: 10.1108/IJCHM-03-2022-0403.
Chen, Y. (2022), “A network analysis of tourist activity”, International Journal of Contemporary Hospitality Management, doi: 10.1108/IJCHM-06-2022-0728.
Csardi, G. and Nepusz, T. (2006), “The igraph software package for complex network research”, International Journal of Complex Systems, p. 1695, available at: www.igraph.org
Feinerer, I. and Hornik, K. (2022), “Tm: Text mining package”, R package version 0.7-9, available at: www.CRAN.R-project.org/package=tm
Ivars-Baidal, J., Casado-Díaz, A.B., Navarro-Ruiz, S. and Fuster-Uguet, M. (2023), “Smart tourism city governance: exploring the impact on stakeholder networks”, International Journal of Contemporary Hospitality Management, doi: 10.1108/IJCHM-03-2022-0322.
Jokar, S., Shojaei, P., Askarifar, K. and Haqbin, A. (2022), “Investigating social risks of construction projects in historic tourism sites in urban districts of developing countries: social network analysis approach”, International Journal of Contemporary Hospitality Management, doi: 10.1108/IJCHM-03-2022-0282.
McLeod, M., Vaughan, D.R., Edwards, J. and Moital, M. (2022), “Knowledge sharing and innovation in open networks of tourism businesses”, International Journal of Contemporary Hospitality Management, doi: 10.1108/IJCHM-03-2022-0326.
Mariani, M. and Baggio, R. (2020), “The relevance of mixed methods for network analysis in tourism and hospitality research”, International Journal of Contemporary Hospitality Management, Vol. 32 No. 4, pp. 1643-1673.
Ness, H., Aarstad, J. and Haugland, S.A. (2022), “Structural networks and dyadic negotiations in tourism destination ecosystems”, International Journal of Contemporary Hospitality Management, doi: 10.1108/IJCHM-03-2022-0309.
Salton, G. and Buckley, C. (1988), “Term-weighting approaches in automatic text retrieval”, Information Processing and Management, Vol. 24 No. 5, pp. 513-523.
Shrestha, R.K., L'Espoir Decosta, J.-N.P. and Shrestha, R. (2023), “Creating value networks through a learning society for indigenous women tourism entrepreneurs”, International Journal of Contemporary Hospitality Management, doi: 10.1108/IJCHM-03-2022-0317.
Vila-Lopez, N. and Kuster-Boluda, I. (2022), “Data mining to reposition a religious tourist destination in COVID-19”, International Journal of Contemporary Hospitality Management, doi: 10.1108/IJCHM-03-2022-0390.
Wang, Y., Jiang, Y., Geng, B., Yan, Z. and Wang, X. (2022), “Social networks, network interaction and resilience of B&bs in rural China”, International Journal of Contemporary Hospitality Management, doi: 10.1108/IJCHM-04-2022-0486.
Wang, Z., Liu, W., Sun, Z. and Zhao, H. (2023), “Understanding the world heritage sites’ brand diffusion and formation via social media: a mixed-method study”, International Journal of Contemporary Hospitality Management, doi: 10.1108/IJCHM-02-2023-0190.
Yang, L., Li, X. and Hernández-Lara, A.-B. (2022), “Scientific collaboration and thematic analysis of the tourism industry in the context of COVID-19: a bibliometric approach”, International Journal of Contemporary Hospitality Management, doi: 10.1108/IJCHM-03-2022-0303.
Further reading
Casanueva, C., Gallego, A. and García-Sánchez, M.-R. (2016), “Social network analysis in tourism”, Current Issues in Tourism, Vol. 19 No. 12, pp. 1190-1209.
Wasserman, S. and Faust, K. (1994), “Social network analysis: methods and applications”, Structural Analysis in the Social Sciences, Cambridge University Press, Vol. 8, pp. 299-302.