Abstract
Purpose
This study analyses agricultural land price dynamics in order to better understand price development and to improve forecast accuracy. Understanding the evolution of agricultural land prices is important when considering sound investment decisions.
Design/methodology/approach
This study applies threshold autoregression to model agricultural land prices. The data includes quarterly observations on Finnish agricultural land prices.
Findings
The study shows that Finnish agricultural land prices exhibit regime-switching behaviour when using past changes in prices as a threshold variable. The threshold autoregressive model not only fits the data better but also improves the accuracy of price forecasts compared to the linear autoregressive model.
Originality/value
The results show that a sharp fall in agricultural land prices temporarily changes the regular development of prices. This information significantly improves the accuracy of price predictions.
Keywords
Citation
Valtiala, J. (2021), "Testing for regime-switching behaviour in Finnish agricultural land prices", Agricultural Finance Review, Vol. 81 No. 2, pp. 292-305. https://doi.org/10.1108/AFR-03-2020-0037
Publisher
:Emerald Publishing Limited
Copyright © 2020, Juho Valtiala
License
Published by Emerald Group Publishing Limited. This article is published under the Creative Commons Attribution (CC BY 4.0) licence. Anyone may reproduce, distribute, translate and create derivative works of this article (for both commercial and non-commercial purposes), subject to full attribution to the original publication and authors. The full terms of this licence may be seen at http://creativecommons.org/licences/by/4.0/legalcode
Introduction
Concerns such as excessive speculative pricing and land grabbing in agricultural land markets have been recently discussed in the European Union (European Commission, 2017). These concerns relate to the long-lasted discussion about the determination of agricultural land prices amongst economists. Models of agricultural land values usually assume that the value is the present value of expected future cash flows, which are appropriately discounted their risk considered. Some studies, however, conclude that prices exhibit bubble behaviour (Roche and McQuinn, 2001; Power and Turvey, 2010). In that case, land prices do not follow their fundamental values as the present value model predicts. Falk and Lee (1998) discovered that land prices deviate from the predictions of present value model in short term, but prices follow market fundamentals in the long run. Similarly, Featherstone et al. (2017) found that Kansas land values slowly adjust to changes in farm income, but they fully respond to the changes in income in the long term. Moss and Katchova (2005) concluded, on the other hand, that agricultural land appears to be consistently over-priced, although it consistently follows the changes in fundamental values. In line with this conclusion, Shi and McCarthy (2013) found that there has been a correspondence between agricultural land price and land rental price development over a long period, but land prices have grown faster than rental prices part of the time.
It appears that the ability of present value model to predict the development of land prices is limited. The results of Kuethe et al. (2014) show that even a comprehensive set of variables may not considerably improve the predictions. Although fundamental values and other exogenous processes explain the past development of prices, their ability to predict the future development is extremely limited, because their own development should be predicted as well. For these reasons, modelling agricultural land prices as a function of prior prices may provide a good alternative when the aim is to approximate current prices and forecast future prices. Furthermore, agricultural land prices appear to exhibit boom and bust cycles in short and intermediate terms (Moss and Katchova, 2005), so the development of prices may follow different regimes under certain conditions. This is usually ignored in the literature on agricultural land prices but may help to explain the price development. Prices may systematically behave differently, for example, when they are falling or when extreme changes occur. Identifying regime-switching behaviour could increase understanding about price development and help to create more accurate forecasts. It should be noted that time frames could also be considered as regimes. In this case, however, predicting the change of the regime is very difficult, and past regimes help to explain very little if at all the current prices and the possible direction of future prices. For this reason, regime switching refers to other types of processes than time frames of different price development in this study.
Modelling agricultural land prices as a function of prior prices has some limitations. Tegene and Kuchler (1994) compared three different models for forecasting agricultural land prices, and they did not recommend using autoregressive integrated moving average (ARIMA) models, which generally lack economic theory and are not sufficiently capable to predict the direction of price changes. They found instead that error correction models with prices and rental rates provided the most accurate forecasts. ARIMA and other non-theoretical models, on the other hand, have the advantage of low data requirements. Non-theoretical models, therefore, are available for making predictions even with the information about prices only. Statistical models provide an alternative for forecasts made by experts. Zakrzewicz et al. (2013) discovered that expert reviews may improve the accuracy of price forecasts. Kuethe and Hubbs (2017), however, found that the experts' forecasts which they analysed were unbiased but inefficient, so that the aggregate forecast error was correlated with past aggregate forecast errors. This shows that expert knowledge does not outperform other methods, but statistical models are also needed. Expert knowledge is regional by nature, which makes it non-generalisable. The question about the future direction of agricultural land prices contains much uncertainty, but the answer is very much wanted. From a farmer's perspective, knowing the future prices would be of great practical value when making investment decisions and for strategic planning (Zakrzewicz et al., 2013). Landowners in general want to anticipate the value changes of their land assets, as the changes may affect their financial position.
This study examines whether Finnish agricultural land prices exhibit regime-switching behaviour. The aim of the study is to increase understanding about the development of agricultural land prices by identifying systematic patterns in the price fluctuation. The results provide valuable information for making investment decisions and predicting the value of current land assets. Regime-switching behaviour in prices does not automatically imply that a regime-switching model would produce more accurate forecasts (e.g. Clements and Krolzig, 1998). For this reason, identifying regime-switching behaviour and forecasting with a regime-switching model are studied separately. The complete list of Finnish agricultural land transactions enables construction of a time series with higher than annual frequency. This increases the number of observations, which is crucial for accurate estimates and forecasts in a time series analysis. The paper proceeds in the following order. Section 2 provides an outlook on Finnish agricultural land markets and presents the data used in the empirical analysis. Section 3 discusses the methods, and Section 4 presents the results and discussion. Section 5 draws conclusions.
Agricultural land markets in Finland and data
Northern location characterises the agriculture and land market in Finland. The northern climate lowers obtainable yields, and soil in general is rocky. Finland has 2.3m ha of agricultural land which is only 6.8% of the total land (Niemi and Väre, 2019). Forests cover most of the land. Barley and oat are the most cultivated grains in Finland, while 0.57 million ha of land is used to produce silage (Niemi and Väre, 2019). Dairy production has a central role in Finnish agriculture and food sector. The number of farms having more than 1 ha of land and receiving farm payments was slightly below 49,500, and the average size of these farms was 45.98 ha (Niemi and Väre, 2019). The average size of land parcels is relatively small, 2.37 ha, and the farm structure is often fragmented, as land parcels are dispersed and sometimes far from the main building (Hiironen and Ettanen, 2012). Farms and agricultural land, however, are unevenly distributed in the country, as most of the farms and agricultural land lie near the shores of Baltic Sea. Soil is generally more fertile closer to the sea, and the Baltics also has a moderating effect on climate. Western Finland is the dominant region in terms of production and agricultural land sales, for the majority of the Finnish agricultural land transactions are made there. The Finnish legislation does not restrict agricultural land sales and ownership, except in some rare cases.
Figure 1 shows the constructed agricultural land price series and real prices for comparison. Median prices started to rapidly decline in early 1990s but grew strongly after the mid-1990s. The down- and upturn coincided with two major economic events. The Finnish economy suffered from a severe recession in the early 1990s. At the same time, Finland started to negotiate to join the European Union (EU), and it became a member in 1995. This implied considerable changes to the Finnish agricultural sector as the whole agricultural policy changed, taking away the old system including fixed producer prices and trade barriers. EU membership did not cause a major financial shock to farms because of economic support in the adjustment period. The economy in general recovered from the recession and started to grow in the mid-1990s. Agricultural land prices rose until the early 2010s. It should be noted that the number of transactions peaked earlier, in 2005, and started to fall until 2010 (Figure 1). The number of transactions has fluctuated approximately at the same level in the 2010s than it did in the 1990s. Prices have fluctuated rapidly in the 2010s, which may indicate increased uncertainty in the agricultural land markets. Interestingly, real prices have fluctuated around the 1990s level during the 2010s. Despite the strong growth in prices since the mid-1990s, the prices in real terms have only occasionally risen above the 1990 level. Anyway, farm profitability has generally worsened during the EU era, making the growth in land prices peculiar. Relatively high level of subsidies and their even distribution between regions might explain this.
The data set includes all the recorded agricultural land transactions in Finland during 1990–2017, and the aggregate quarterly time series is constructed from it. The data originate from the National Land Survey of Finland (2017). They are not openly available but were obtained for research purposes. The data include only transactions between non-relatives and purchases of more than 2 ha of agricultural land. The quarterly frequency seemed most reasonable when optimising between the number of transactions in a period and the total number of observations in the final series. The series was constructed by first stacking all the transactions during a quarter and then taking the median value without selecting or adjusting the observations. Because each transaction is unique in terms of characteristics the land possesses and motives that buyers and sellers have, prices per hectare exhibit huge variation. For this reason, similar studies often regress the price on the characteristics of a land parcel to obtain estimated prices with qualitative differences removed. The data of this study was not enough to implement a proper hedonic pricing analysis. Despite this shortcoming, the median price follows a clear trend, and the median naturally ruled out extremely high and low prices which frequently occurred. Even though the number of transactions differs between quarters, 157 transactions were carried out on average during a quarter, the minimum being 52. Table 1 shows the summary statistics of agricultural land prices.
The average number of transactions systematically varied between quarters. Most of the transactions were made in the second and fourth quarter, whereas the average number of transactions was lowest in the third quarter. Because the number of transactions exhibited seasonality, the prices were also inspected for a regular seasonal pattern. Lags 4, 8 and 12 in the autocorrelation function (ACF) indeed had a spike, which could indicate seasonality during some periods (Figure A1 in Appendix). Further examination of the AF, however, showed that regular seasonality started quite recently, after 2012. No regular seasonality seems to exist before that, but price levels frequently dipped in the third quarter after 2012. Seasonality causes autocorrelated residuals if left unaddressed, but regular seasonality does not appear consistently throughout the series. The model, therefore, did not require any seasonal adjustments.
Methods
Several methods to model regime-switching behaviour exist. Regime-switching models have several applications ranging from agricultural commodity markets (Asche et al., 2013; Liu et al., 2013) to oil prices (Zhang and Zhang, 2015; de Albuquerquemello et al., 2018). Prices of various goods and products have been shown to exhibit regime-switching behaviour, and, therefore, agricultural land prices unlikely make an exception. Some models assume that the underlying process, or the state variable in other words, causing regime switches is observable while some assume an unobservable process. Models also differ in their assumptions on the speed and probability of regime switches. Threshold autoregressive (TAR) models assume an instant and certain switch when the switching condition is met, while smooth transition autoregressive models assume gradual switches between regimes. Simple Markov-switching models, on the other hand, assume that an unobservable process causes regime switches which are stochastic. This implies that the regime switches with a certain probability. Franses and van Dijk (2000) and Enders (2015), among others, provide an introduction to different regime-switching models.
Studies have shown that regime-switching models do not outperform linear models in forecasting, and this result applies to regime-switching models more generally (Clements and Krolzig, 1998; Clements et al., 2003). For this reason, the most appropriate regime-switching model cannot be selected based on forecasting performance. In the context of this study, self-exciting threshold autoregressive (SETAR) model seemed an appropriate choice. It provides a straightforward way to test for regime switching in agricultural land prices, and the results are easy to interpret and practitioners can easily apply them. Lizieri and Satchell (1997) also argue that observable state variable makes SETAR preferable to Markov-switching model. The fact that the state variable is observable in SETAR indeed has a great practical value. This relates to the comprehensibility and applicability of the results, which is an important aspect of this study.
SETAR models assume that a time series develops conditionally on the current regime. Self-exciting TAR (SETAR) models use a lag
The error term
Hansen (1997) discusses the heteroscedastic errors in the SETAR model and shows that the iid assumption can be relieved. It comes at the cost of more complicated estimation, however. The parameters
Hansen's supremum test (1996) enables testing for regime-switching behaviour in a series. A linear autoregressive (AR) model is a special case of SETAR, but with estimated
Non-linearity complicates forecasting with a SETAR model after a one-step ahead forecast
Equation (2) presents how to obtain an MC estimate for
The empirical analysis of this study proceeds by first testing the series for unit roots to determine the number of differences needed. The next step determines an adequate linear AR model. Then the analysis proceeds to testing whether the development of Finnish agricultural land prices exhibits regime-switching behaviour with Hansen's test. If any threshold variable provides significantly lower SSR than the linear AR model, it follows that the development of prices follows some non-linear process with a threshold
Results
The analysis began by testing the price series for stationarity. Investigating stationarity essentially shows the order of integration needed. Non-stationarity produces meaningless results if not correctly addressed, but too many differences cause loss of information and complicate the interpretation of results. If the series proves non-stationary, taking one or more differences makes it stationary. Table A1 in Appendix shows unit-root test results. The ACF and the results from unit-root tests indicate the presence of a unit root in the agricultural land price series. Differencing made the series stationary as the ACF of the differenced series shows (Figure A1 in Appendix). Log-transformation also became necessary to achieve residual normality. The resulting log-differences are approximately equal to percentage changes, and they were used in further analysis. Including five lags in the model eliminated residual autocorrelation, so the basic AR model and also the SETAR model had an AR order of 5. Table A2 in Appendix shows the parameter estimates and diagnostics of the AR model.
Testing for regime-switching behaviour involved searching for the most appropriate threshold variable. The testing procedure searched for the best-fitting specification over lags 1–5 and additionally for the annual difference as in Hansen (1997). About 3,000 bootstrap draws formed the empirical distribution for the test statistic, and a trimming percentage of 15 was applied. The annual difference shows the average growth during a year, and Hansen found this type of threshold variable superior over single lags. Table 2 presents the results from Hansen's test. The results clearly show that the SETAR model fits the data significantly better than the linear AR model when using the fourth lag as the threshold variable. The third lag also slightly exceeded the linear model in explanatory power, but the SETAR model fitted the data worse than the linear model when using other variables. Because the test revealed regime-switching behaviour with the fourth lag as the threshold variable, the analysis proceeded to estimate SETAR.
Table 3 shows the estimated parameters and additional statistics of the SETAR model. The heteroscedasticity test gave no indication of heteroscedastic errors, and further adjustments for parameters therefore became unnecessary. The SETAR model fitted the data significantly better than the AR model, as the threshold model raised the coefficient of determination from 0.362 in the AR model to 0.518. The estimated
The value of
Figure 3 illustrates the appearance of different regimes, crosses representing regime uncertainty, circles regime 1 and triangles regime 2. The number of observations was relatively low in the first regime, and the appearances of the regime remained very short-lived. The extreme events of sharp decreases in prices happen rarely, so the regime 1 does not persist very long at a time. The variation of prices has become wider in the 2010s, which coincides with the fact that the first regime appears most often during the period. This implies that SETAR helps to model such uncertainty. Regime uncertainty also appeared relatively often, which could be considered as a weakness of the model.
The analysis also compared the forecasting performance of the AR and SETAR models. Although the SETAR model fitted the data significantly better, it does not automatically imply more accurate forecasts compared with the AR model (Clements and Smith, 1997; Franses and van Dijk, 2000). The approximate normality of residuals (Table 3) made the use of MC feasible in SETAR forecasts, and the point estimates of forecasts were formed from the sample of 3,000 MC draws. The forecast period spanned from the first quarter of 2016 to the fourth quarter of 2017, being a dynamic out-of-sample forecast. Forecasting applied parameter estimates from an auxiliary model estimated using a 1990–2015 sample.
The results of this auxiliary model differed from the full-sample model. This was mostly due to the fact that the regime 1 mostly appeared during the 2010s. The threshold parameter of the auxiliary model had much wider confidence interval spanning from −0.163 to −0.072 with a point estimate of −0.148, but trimming restricted the value to the original −0.091. Trimming was extremely important in this case, because the first regime captured extreme behaviour. The threshold value defines the border between extreme and conventional, and narrowing the extreme further would have made the solution completely trivial. Using the conventional trimming percentage of 15 (Hansen, 1996) provided the optimal
Table 4 presents the results for eight forecast estimates, actual values, two forecast accuracy measures. The SETAR model performed better in this case having both forecast root mean square error and mean absolute error lower than in the AR model. The SETAR model predicted the direction of change correctly in six cases out of eight. Figure 4 further illustrates that the SETAR model captured swings in prices better, whereas the AR estimates exhibited much less fluctuation. This forms a considerable qualitative advantage for SETAR given the wide variation in prices during recent years.
Conclusions
The analysis provided new information about the feasibility of SETAR in modelling and forecasting agricultural land prices. The test showed that Finnish agricultural land prices exhibit regime-switching behaviour, as the SETAR model fitted the price data significantly better than the AR model. The model detected that the prices develop differently a year after a sharp decrease in prices. The ability of SETAR to distinguish extreme events from the conventional variation brings flexibility to modelling. This attribute helped to capture the price volatility during the 2010s better than the AR model. It also made the model forecast more accurately than the AR model. To conclude these findings, SETAR provides a useful framework to model and forecast agricultural land prices. The flexibility of TAR brings in additional questions to analyse. The regime switches considered in this study were endogenous and observable, but other options also exist. Some exogenous process could cause the switches, or the switches could be unobservable as Markov-switching models assume. It could be asked whether different drivers such as farm profitability or macroeconomic factors affect land prices differently during different regimes. These are left for future research.
The regimes, however, lacked further qualitative interpretation. This study statistically proved the regime-switching behaviour, but it could not explain what causes sharp decreases in prices. This coincides with the critique presented by Tegene and Kuchler (1994). The study shows that agricultural land prices may exhibit regime-switching behaviour, which should be considered when studying agricultural land markets in other countries. Federal Reserve Banks, for example, quarterly publish percentage changes in farmland values. After the first difference and logarithmic transformations, the data of this study were approximately percentage changes, thus similar analysis could easily be implemented elsewhere. Based on these particular results, it is advisable to buy agricultural land while the prices fluctuate modestly or increase slightly. This maximises the probability that the prices develop steadily also in the near future.
Figures
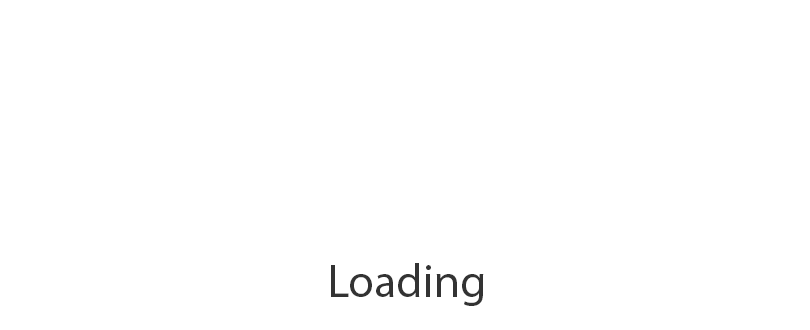
Figure 1
Median agricultural land prices and number of transactions in Finland 1990–2017. Finnish gross domestic product deflator (Statistics Finland, 2019) used to calculate real prices
Summary statistics of agricultural land prices in Finland for 1990–2017
Nominal | Real (in 2010 prices) | |
---|---|---|
Average change (€) | 20.41 | −5.22 |
Standard deviation of change (€) | 695.36 | 674.09 |
Minimum change (€) | −1,988.52 | −1,783.33 |
Maximum change (€) | 2,464.83 | 2,213.06 |
Lowest price level (€) | 2,328.76 | 2,911.86 |
Highest price level (€) | 10,000.00 | 8,909.70 |
Quarterly statistics | ||
Average number of transactions in quarter | 157 | |
Lowest number of transactions in quarter | 52 | |
Highest number of transactions in quarter | 327 | |
Average number of transactions in first quarter | 154 | |
Average number of transactions in second quarter | 199 | |
Average number of transactions in third quarter | 98 | |
Average number of transactions in fourth quarter | 178 |
Estimated threshold parameters, F-test values and respective p-values from testing the threshold model against the linear model
Variable | Threshold parameter | F-test value | P-value |
---|---|---|---|
−0.093 | 22.25 | 0.075 | |
−0.062 | 11.27 | 0.65 | |
0.068 | 24.06 | 0.05 | |
−0.091 | 34.13 | 0.004 | |
−0.064 | 20.65 | 0.102 | |
−0.089 | 10.37 | 0.767 |
Parameter estimates and diagnostics of the SETAR model
Parameter | Estimate | Standard error | 95% confidence interval | |
---|---|---|---|---|
−0.091 | [−0.093, −0.041] | |||
Regime 1: | ||||
Constant | 0.037 | 0.066 | [ −0.218, 0.197] | |
0.064 | 0.227 | [ −0.593, 0.655] | ||
−0.063 | 0.218 | [ −0.735, 0.462] | ||
−0.448 | 0.184 | [ −1.011, −0.006] | ||
0.401 | 0.458 | [ −1.275, 1.5] | ||
0.441 | 0.158 | [0.03, 0.862] | ||
Regime 2: | ||||
Constant | 0.007 | 0.01 | [ −0.027, 0.029] | |
−0.624 | 0.098 | [ −0.843, −0.407] | ||
−0.164 | 0.106 | [ −0.383, 0.123] | ||
0.127 | 0.114 | [ −0.122, 0.388] | ||
0.369 | 0.135 | [0.055, 0.786] | ||
0.211 | 0.109 | [ −0.047, 0.453] | ||
White heteroscedasticity test p-value: 0.908 |
Shapiro–Wilk normality test p-value: 0.067R2: 0.518
Forecast point estimates and mean squared errors
Time | SETAR | AR | Observed |
---|---|---|---|
2016q1 | 0.096 | −0.062 | 0.137 |
2016q2 | −0.102 | 0.043 | −0.163 |
2016q3 | −0.172 | −0.097 | −0.093 |
2016q4 | 0.193 | 0.097 | 0.104 |
2017q1 | −0.009 | −0.003 | 0.022 |
2017q2 | 0.148 | 0.002 | −0.072 |
2017q3 | −0.14 | −0.029 | −0.233 |
2017q4 | 0.108 | 0.039 | 0.115 |
Forecast RMSE | 0.099 | 0.13 | |
Mean absolute error | 0.078 | 0.099 |
Note(s): AR = autoregressive model, SETAR = selfexciting threshold autoregressive model, RMSE = root mean square error
Results from unit-root tests
ADF (with intercept) | PP (with intercept) | ERS (with intercept) | ERS (with intercept and trend) | |
---|---|---|---|---|
Test statistic | −0.54 (4 lags) | −1.53 (4 lags) | −0.587 (4 lags) | −1.12 (4 lags) |
P-value | >0.1 | >0.1 | >0.1 | >0.1 |
Note(s): ADF = augmented Dickey–Fuller test, PP = Phillips–Perron test, ERS = Elliott–Rothenberg–Stock test
Estimation results and diagnostics of the autoregressive model
Variable | Coefficient | Standard error (p-value in parentheses) |
---|---|---|
Constant | 0.003 | 0.009 (0.729) |
−0.523 | 0.096 (<0.001) | |
−0.203 | 0.104 (0.053) | |
−0.047 | 0.106 (0.663) | |
0.432 | 0.105 (<0.001) | |
0.3 | 0.099 (0.003) | |
Portmanteau serial correlation test p-value: 0.729 (1 lag) | ||
0.932 (2 lags) | ||
0.989 (4 lags) | ||
0.985 (8 lags) | ||
0.687 (12 lags) | ||
0.929 (24 lags) | ||
White heteroscedasticity test p-value: 0.869 |
Normality test p-value: 0.281R2: 0.362
Appendix
References
Asche, F., Oglend, A. and Tveteras, S. (2013), Regime shifts in the fish meal/soybean meal price ratio, Journal of Agricultural Economics, Vol. 64 No. 1, pp. 97-111, doi: 10.1111/j.1477-9552.2012.00357.x.
Clements, M.P. and Krolzig, H. (1998), “A comparison of the forecast performance of markov‐switching and threshold autoregressive models of US GNP”, Econometrics Journal, Vol. 1 No. 1, pp. 47-75, available at: http://doi-org.libproxy.helsinki.fi/10.1111/1368-423X.11004.
Clements, M.P. and Smith, J. (1997), “The performance of alternative forecasting methods for SETAR models”, International Journal of Forecasting, Vol. 13 No. 4, pp. 462-475, doi: 10.1016/S0169-2070(97)00017-4.
Clements, M., Franses, P. and Smith, J. (2003), “On SETAR Non-linearity and forecasting”, Journal of Forecasting, Vol. 22 No. 5, pp. 359-375, available at: http://doi-org.libproxy.helsinki.fi/10.1002/for.863.
de Albuquerquemello, V.P., de Medeiros, R.K., Da Nóbrega Besarria, C. and Maia, S.F. (2018), “Forecasting crude oil price: does exist an optimal econometric model?”, Energy, Vol. 155, pp. 578-591, doi: 10.1016/j.energy.2018.04.187.
Enders, W. (2015), Applied Econometric Time Series, 4th ed., John Wiley and Sons, Hoboken, NJ.
European Commission (2017), “Sales of farmland: commission issues guidelines to member states”, available at: https://ec.europa.eu/commission/presscorner/detail/en/IP_17_3901 (accessed 19 May 2020).
Falk, B. and Lee, B. (1998), “Fads versus fundamentals in farmland prices”, American Journal of Agricultural Economics, Vol. 80 No. 4, pp. 696-707, available at: http://doi-org.libproxy.helsinki.fi/10.2307/1244057.
Featherstone, A.M., Taylor, M.R. and Gibson, H. (2017), “Forecasting Kansas land values using net farm income”, Agricultural Finance Review, Vol. 77 No. 1, pp. 137-152, doi: 10.1108/AFR-03-2016-0025.
Franses, P.H. and van Dijk, D. (2000), Non-linear Time Series Models in Empirical Finance, Cambridge University Press, Cambridge, doi: 10.1017/CBO9780511754067.
Hansen, B.E. (1996), “Inference when a nuisance parameter is not identified under the null hypothesis”, Econometrica, Vol. 64 No. 2, pp. 413-430, available at: https://www.jstor.org/stable/2171789.
Hansen, B.E. (1997), “Inference in TAR models”, Studies in Nonlinear Dynamics and Econometrics, Vol. 2 No. 1, doi: 10.2202/1558-3708.1024.
Hansen, B.E. (2000), “Sample splitting and threshold estimation”, Econometrica, Vol. 68 No. 3, pp. 575-603, doi: 10.1111/1468-0262.00124.
Hansen, B.E. (n.d.), Inference in TAR Models [R Programs and Data], University of Wisconsin–Madison, Madison, WI, available at: https://www.ssc.wisc.edu/∼bhansen/progs/snde_97.html.
Hiironen, J. and Ettanen, S. (2012), Peltoalueiden tilusrakenne ja sen parantamismahdollisuudet (in Finnish), National Land Survey of Finland, Helsinki, available at: https://www.maanmittauslaitos.fi/sites/maanmittauslaitos.fi/files/old/Peltoalueiden%2520tilusrakenne%2520ja%2520sen%2520parantamismahdollisuudet.pdf.
Kuethe, T.H. and Hubbs, T. (2017), “Bankers' forecasts of farmland values: a qualitative and quantitative evaluation”, Journal of Agricultural and Applied Economics, Vol. 49 No. 4, pp. 617-633, doi: 10.1017/aae.2017.16.
Kuethe, T.H., Hubbs, T. and Morehart, M. (2014), “Farmland returns and economic conditions: a FAVAR approach”, Empirical Economics, Vol. 47, pp. 129-142, doi: 10.1007/s00181-013-0730-5.
Lin, J.‐L. and Granger, C.W.J. (1994), “Forecasting from non‐linear models in practice”, Journal of Forecasting, Vol. 13, No. 1 pp. 1-9, doi: 10.1002/for.3980130102.
Liu, X., Filler, G. and Odening, M. (2013), “Testing for speculative bubbles in agricultural commodity prices: a regime switching approach”, Agricultural Finance Review, Vol. 73 No. 1, pp. 179-200, doi: 10.1108/00021461311321384.
Lizieri, C. and Satchell, S. (1997), “Property company performance and real interest rates: a regime-switching approach”, Journal of Property Research, Vol. 14 No. 2, pp. 85-97, doi: 10.1080/095999197368654.
Moss, C.B. and Katchova, A.L. (2005), “Farmland valuation and asset performance”, Agricultural Finance Review, Vol. 65 No. 2, pp. 119-130, doi: 10.1108/00214660580001168.
National Land Survey of Finland (2017), Official Purchase Price Register Data on Agricultural Land Transactions 1990-2017, National Land Survey of Finland, Helsinki.
Niemi, J. and Väre, M. (2019), “Agriculture and food sector in Finland 2019”, Natural Resources Institute Finland (Luke), Helsinki, available at: http://urn.fi/URN, ISBN: 978-952-326-771-8.
Pfaff, B. (2008), Analysis of Integrated and Cointegrated Time Series with R, 2nd ed., Springer, New York, NY.
Power, G.J. and Turvey, C.G. (2010), “US rural land value bubbles”, Applied Economics Letters, Vol. 17 No. 7, pp. 649-656, doi: 10.1080/13504850802297970.
R Core Team (2019), R (3.6.2 (2019-12-12)) [A Language and Environment for Statistical Computing], R Foundation for Statistical Computing, Vienna.
Roche, M.J. and McQuinn, K. (2001), “Testing for speculation in agricultural land in Ireland”, European Review of Agricultural Economics, Vol. 28 No. 2, pp. 95-115, doi: 10.1093/erae/28.2.95.
Shi, S. and McCarthy, I. (2013), “Pricing of New Zealand dairy farmland”, Journal of Property Investment and Finance, Vol. 31 No. 2, pp. 118-134, doi: 10.1108/14635781311302564.
Statistics Finland (2019), “Gross domestic product and national income, supply and demand, quarterly, 1990Q1-2019Q3”, Statistics Finland's PxWeb databases, available at: http://pxnet2.stat.fi/PXWeb/pxweb/en/StatFin/StatFin__kan__ntp/statfin_ntp_pxt_11xa.px/ (accessed 15 March 2020).
Tegene, A. and Kuchler, F. (1994), “Evaluating forecasting models of farmland prices”, International Journal of Forecasting, Vol. 10 No. 1, pp. 65-80, doi: 10.1016/0169-2070(94)90051-5.
Zakrzewicz, C.J., Brorsen, B.W. and Briggeman, B.C. (2013), “Accuracy of qualitative forecasts of farmland values from the federal reserve's land value survey”, Journal of Agricultural and Applied Economics, Vol. 45 No. 1, pp. 159-170, doi: 10.1017/S1074070800004648.
Zhang, Y. and Zhang, L. (2015), “Interpreting the crude oil price movements: evidence from the Markov regime switching model”, Applied Energy, Vol. 143, pp. 96-109, doi: 10.1016/j.apenergy.2015.01.005.
Acknowledgements
This work was supported by the Ministry of Agriculture and Forestry of Finland [CAPMAP, 2017 -2020].Declaration of interest: The Ministry of Agriculture and Forestry of Finland did not participate, nor otherwise affected the research and publication process. I have no conflict of interest to declare.